Blog
Can Machine Learning Lead to Valuable Business Insights?
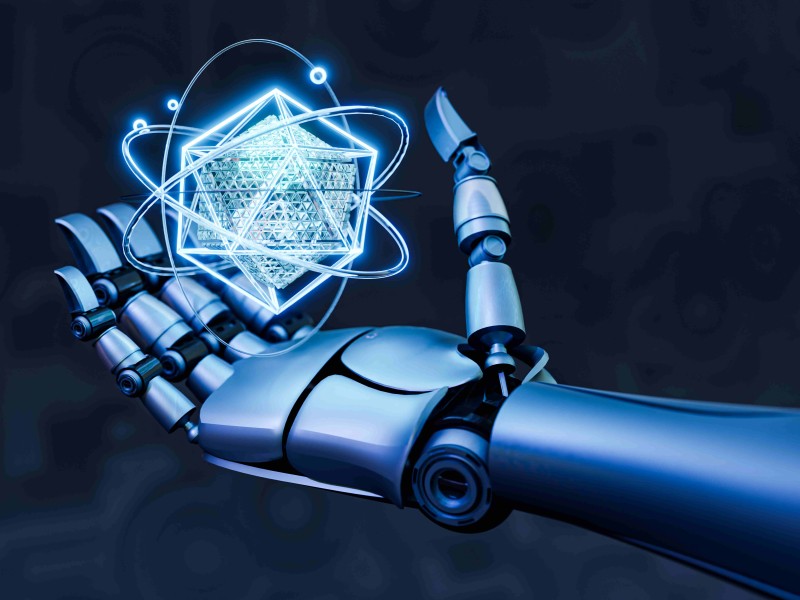
Expanded technology usage and accessibility have driven the convergence of various digital technologies and resulted in rapid technological advancement and transformation. This event conglomeration is more commonly called the Fourth Industrial Revolution. This revolution is founded around the fusion of innovations such as the Internet of Things, robotics, big data, artificial intelligence, and cloud computing, which influence various world sectors. These sectors are influenced by the Industrial Revolution's potential to increase productivity, economic growth, teaching ability, and the rate at which data is generated and used (Skilton & Hovsepian, 2017).
Most industries and companies generate vast amounts of data across each of their divisions and never use the data they produce to improve their decision-making. This is generally because most datasets are highly intricate, complex to extract or transform, require real-time processing, or possess a significant number of dimensions. Consequently, analysing such datasets for decision-making purposes can take business time and effort.
Machine learning algorithms are a solution and can significantly assist with decision-making from datasets by producing predictive models and providing valuable insights. These algorithms, a subset of artificial intelligence, are necessary to guarantee optimal and accurate decision-making with large and small datasets across any industry or business.
As depicted in Figure 1.1, the machine learning process generally follows an outline of the following steps: data collection, pre-processing, feature selection, algorithm selection, model training, model evaluation, and deployment. Machine learning can be applied to many problems, such as classification, regression, clustering, pattern recognition, association, and dimensionality reduction (Flach, 2012).
As Mitchell (1980) described, learning is taking past experiences and generalising from them to deal with new situations related to past experiences. Therefore, machine learning (ML) involves algorithms that learn from experience through historical data and can predict what can happen next based on the learning from the historical data. The difference between an algorithm and a predictive model is that a specific ML algorithm trains a model; after being trained, a model is then used for predictions (Danks, 2014). ML algorithms search through an 𝑛-dimensional space of a given dataset to find an acceptable generalisation or representation of the data. A fundamental task of ML is inductive machine learning, which involves input-output mapping or the estimation of the dependencies between inputs and outputs. The mapping can be formalised using different functions or techniques and is derived from limited data samples, known as training data, to form a generalisation. Once a sufficient generalisation is formed, predictions can be made for valuable and insightful decision-making to help improve the business.
References
Skilton, M., & Hovsepian, F. (2017). The 4th Industrial Revolution: Responding to the Impact of Artificial Intelligence on Business. Palgrave Macmillan.
Flach, P. (2012). Machine Learning: The Art and Science of Algorithms that Make Sense of Data. Cambridge University Press.
Okun, O., & Valentini, G. (2011). Ensemble Methods in Machine Learning. Springer.
Mitchell, T. M. (1980). The Need for Biases in Learning Generalisations (Technical Report CBM-TR-117). Rutgers University.
Danks, D. (2014). Unifying the Mind: Cognitive Representations as Graphical Models. MIT Press.
For enquiries, please contact This email address is being protected from spambots. You need JavaScript enabled to view it..
This article was written by F.Conradie