Blog
What is ML-based monitoring and alerting?
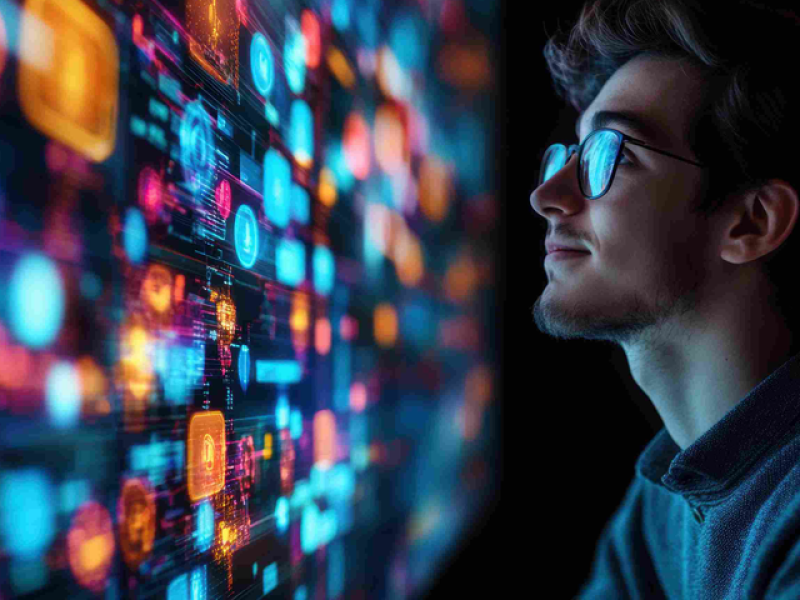
Machine learning (ML) has significantly evolved since its conception in the 1950’s. One of the minds behind the technology and field of study was Alan Turing, a computer scientist and artificial intelligence (AI) pioneer. Turing famously challenged the expectations and limitations of computers by posing the question, “Can machines think?”
Decades later, the power of AI and ML is still being unpacked. Putting all the philosophical banter aside, ML capabilities have proven invaluable in IT security, especially when it comes to monitoring and alerting.
In this blog, you will explore the differences between traditional IT monitoring and ML-based monitoring, the benefits of ML-based monitoring and alerting, how to choose the right ML-based monitoring and alerting tools, and best practices for protecting sensitive data.
ML-based monitoring and alerting explained
ML-based monitoring and alerting takes a modern approach to monitoring and tracking the performance of a system or application using ML models. ML-based monitoring enables IT professionals to collect and analyze large amounts of data in real time to identify patterns and anomalies that could indicate potential issues or failures.
The goal of ML-based and anomaly-based monitoring: proactively detect and address issues before they get the chance to impact a system’s performance or availability.
So, does this mean that ML-based monitoring can predict the future? Not exactly. ML-based monitoring tools have limitations like any other technology. It uses data to identify anomalies and ensure accuracy over time. But anomaly-based monitoring isn’t immune to unpredictable and unexpected changes that are bound to happen. Outliers and unforeseen events such as COVID-19 can cause data to deviate and make predictions less realistic — but that’s a challenge with any tool. However, by and large, the benefits outweigh the potential drawbacks.
Traditional monitoring versus ML
In traditional monitoring, the collection and analysis of data relies on human intervention. IT experts comb through the data to track performance or identify potential issues. This is incredibly time consuming and labor intensive, which uses predetermined metrics and thresholds set by these experts to flag any abnormalities.
On the other hand, ML is a form of artificial intelligence that empowers computer systems to automatically learn and improve from data without being explicitly programmed. In ML, algorithms are trained on large datasets to recognize patterns and make predictions. This enables the system to adapt and improve its monitoring capabilities over time, without the need for human intervention.
Unlike traditional monitoring, which relies on predefined rules and thresholds, ML can identify anomalies and patterns that may not have been previously considered by human experts, often making it more efficient and accurate.
Taking a closer look at threshold-based monitoring limitations
In any case, traditional monitoring that uses a threshold-based approach has few drawbacks. One major concern is that it can generate a lot of false positives, which can contribute to alert fatigue and decrease overall trust in the monitoring system. When the threshold is set too low, alerts are triggered in a frenzy following even the most minor or insignificant changes.
Imagine the smoke detector in your home. You fry an egg over the stove, light a candle or pop a slice of bread in the toaster. In each instance, the smoke detector sounds the alarm and confuses even the smallest amounts of smoke as a five-alarm house fire. Over time, you become desensitized to the noise and begin to ignore the blaring sounds in your everyday life. This could possibly cause you to ignore a legitimate housefire.
It’s a simple analogy that can be applied to system administrators and IT professionals who may become desensitized to traditional alerts and miss critical issues. Additionally, traditional static threshold monitoring struggles to detect anomalies in dynamic systems, where performance fluctuates constantly. This can lead to missed anomalies and delayed responses, potentially causing significant downtime or performance issues. Therefore, while traditional static threshold monitoring has its benefits, it is important to consider these limitations and supplement it with other monitoring techniques for a more comprehensive approach.
An introduction to ML-based monitoring and alerting
ML-based monitoring may sound intimidating to new IT professionals, managed service providers (MSPs) and business owners. But the benefits are critical. ML-based monitoring is a more advanced approach that utilizes algorithms and historical data to identify patterns and anomalies in system performance.
This allows for more accurate and proactive alerting, as well as the ability to adapt to changing environments and usage patterns. By incorporating ML-based monitoring into your overall monitoring strategy, you can reduce false alarms and quickly identify and resolve issues before they escalate. How does ML-based monitoring and alerting work?
ML-based monitoring helps to automatically define the most optimal threshold. Until now, the task of manually configuring thresholds was labor intensive and, by and large, required an experienced engineer to carry out.
ML-based monitoring and alerting works by utilizing ML algorithms to analyze large amounts of data in real time and identify patterns and anomalies that may indicate potential issues or errors. This process involves several steps, starting with data collection and feature engineering. The first step is to identify relevant metrics and system data that will be used for analysis. This can include metrics such as response time, CPU usage and error logs. Once the data is collected, it is preprocessed and transformed to make it suitable for input into ML models.
Next, the data is fed into ML models, which use various algorithms to identify patterns and anomalies. These models can be trained on historical data to learn what normal behavior looks like and can then detect any deviations from this normal behavior. If an anomaly is detected, an alert is triggered, and appropriate actions can be taken to address the issue. ML-based monitoring and alerting systems can also continuously learn and adapt to changing patterns and behaviors, making them more effective over time. This enables proactive monitoring and detection of potential issues, reducing downtime and improving overall system performance.
With ML-based monitoring, you can define a more optimal threshold based on advanced analytical techniques. You no longer require highly skilled expertise to define the optimum threshold, so senior-level technicians are free to prioritize more pressing priorities.
Building and training ML models for monitoring
Think about training ML models and it can feel daunting. However, building and training them for monitoring is a crucial aspect of maintaining a successful and efficient monitoring system. One of the main considerations when building these models is the choice between supervised and unsupervised learning techniques for anomaly detection.
Supervised learning involves providing the model with labeled data to learn from, while unsupervised learning allows the model to detect anomalies on its own based on patterns and trends in the data. Both approaches have their own advantages and disadvantages, and the choice ultimately depends on the specific needs and requirements of the monitoring system.
Additionally, continuous model training and improvement over time is essential for ensuring the accuracy of the ML models. As the data and environment change, the models need to be retrained and fine-tuned in order to adapt and accurately detect anomalies. This ongoing training process helps to continuously improve the performance of the models and ensure the reliability of the monitoring system.
Alerting mechanisms and notification systems
Setting dynamic thresholds based on historical data and learned patterns is an important aspect of alert management. By analyzing past data and patterns, organizations can determine the appropriate threshold levels for their alerts. This approach allows for a more accurate and efficient alert system, as the thresholds are tailored to the specific needs and trends of the organization.
Furthermore, prioritizing alerts based on severity and potential impact is crucial in ensuring that the most critical issues are addressed promptly. This approach ensures that resources are allocated to the most urgent alerts, minimizing the risk of potential damage. By combining these strategies, organizations can effectively manage their alerts and proactively address potential issues.
Benefits of implementing ML-based monitoring and alerting
As an IT leader, business owner or MSP professional, you always want to be efficient and save resources when you can. There are several benefits of ML-based monitoring and alerting that can be incredibly helpful and valuable to organizations.
The benefits include:
- Proactive and more accurate issue detection and minimized downtime.
- Time saved that would otherwise be spent on threshold configurations.
- Reduced alert fatigue and increased efficiency.
- Enhanced visibility into complex IT systems.
Have you ever wondered why your computer is suddenly slow? Often, a sign of malware includes performance data that deviates from normal patterns. ML-based monitoring is essential to picking up on these deviations. To tap into these benefits, you’ll need to choose the right solutions suitable for your unique needs.
How to choose the right ML-based monitoring tools and platforms for your needs
You search for an ML-based monitoring and alerting solution and you’re wondering what you should look for. Integration is a key consideration that comes to mind:
Integration with existing monitoring and ticketing systems
When selecting an ML-based monitoring and alerting solution, it is important to consider its integration with existing monitoring and ticketing systems. This integration enables a seamless flow of data and alerts between the different systems, reducing the risk of information being missed or lost. It also enables the ML-based solution to leverage existing data and insights from the monitoring and ticketing systems, leading to more accurate and efficient alerts. Additionally, integration with existing systems can streamline the process of creating and managing tickets, ensuring prompt resolution of issues. Ultimately, choosing a solution that integrates well with existing systems can improve the overall effectiveness and efficiency of the monitoring and alerting process.
Security and data privacy best practices for ML-based monitoring and alerting
When using ML-based monitoring and alerting tools, it is important to consider both security and data privacy to keep your data safe. One way to protect sensitive data used for training and monitoring is by implementing strong data encryption techniques. This will ensure that even if the data is breached, it cannot be accessed and used by unauthorized parties.
Another important aspect to consider is data privacy. It is crucial to comply with data privacy regulations, such as GDPR and CCPA, when collecting and storing data for ML-based monitoring and alerting. This includes obtaining consent from users before collecting their data and implementing strict data retention policies.
In addition to these measures, it is also important to constantly update and monitor the ML algorithms used in these tools to ensure they are not biased and do not compromise data privacy. As the capabilities of ML-based monitoring and alerting tools continue to evolve, it is crucial to stay updated on the latest advancements and implement them in a responsible manner to protect sensitive data. This includes utilizing unsupervised learning for root cause analysis and incorporating predictive maintenance and self-healing capabilities to improve IT systems' security and data privacy. By staying vigilant and implementing these measures, we can ensure that our data remains safe while using ML-based monitoring and alerting tools.
The promise of ML-based monitoring and alerting
ML-based monitoring and alerting provides numerous benefits, including improved efficiency, increased accuracy and proactive issue detection. It can help IT professionals and businesses to better maintain and protect their IT infrastructure, leading to improved performance and reduced downtime. Additionally, with the continuous advancement and development of ML technology, the future of monitoring and alerting looks even more promising.
Acronis Cyber Protect Cloud with Acronis Management (RMM) is a natively integrated solution that leverages ML-based monitoring and alerting. Acronis ML-based monitoring and smart alerting enables MSPs to proactively respond to developing and sudden changes in their client IT environments before a problem escalates. The feature also empowers their technicians with enhanced accuracy of anomaly detection, alerting and automatic threat remediation. The solution empowers IT professionals of all experience levels by simplifying remote monitoring, management and protection of client endpoints.