Case Studies
Warehouse Slotting Optimisation Case Study – DSV
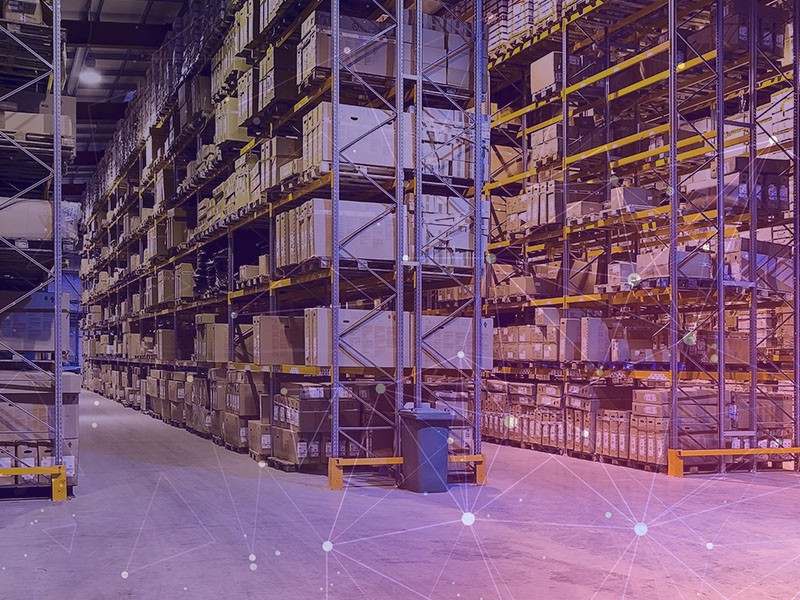
Main Objective
The main objective was to ensure the implemented slotting methodology results in the most efficient and effective process and that daily picking targets will be met.
The comparative model report gave the client a good indication of the units per hour that can be achieved. Different scenarios were explored and tested to get to the best possible scenario.
The model’s key objective is to determine the optimal slotting strategy by testing various scenarios that best fit, to determine the optimal slotting strategy by testing various scenarios that best fit the operation’s objectives, that will meet the daily target.
Parameters
The following elements were considered by the Optislot® optimisation software:
- Daily picking target (picking productivity)
- Congestion and damages
- Order quality
- Warehouse utilisation
- Order fulfilment
- Minimise replenishments
- Improved decision making
- Continuous improvement initiatives
APPROACH
DSV Case Study - Optislot®
Figure 1: Optislot® images of the warehouse heatmap
The slotting optimisation model was used to run various slotting strategies to establish the best slotting strategy that would help DSV achieve daily picking targets without compromising order quality.
The importance of allocating the correct amount of pick faces (product) per SKU to avoid replenishment during picking activities was key to developing the optimal slotting strategy.
Tasks Completed
The following tasks were completed during the development process:
- Data collection, scrubbing, and analysis
- Validation of operating parameters
- Warehouse setup within Optislot®
- Slotting strategies development
- Optislot® configuration to align with agreed-upon strategies
- Consider historical order data
- Generation of comparative scenarios
- Generate comparative reports per scenario
- Analysis of scenarios and recommendations
- Generation of moves list for implementation purposes
- Consider future order data for 2021
RESULTS
Six warehousing strategies were identified:
Scenario 1a - Increased picking productivity based on a pick path sequence goal (limited to one pick face per SKU).
This scenario focussed on reducing horizontal travel distance by slotting items according to a hit sequence goal (unit movement). This scenario does not take congestion into account but will keep horizontal travel distance to a minimum. Only one pick face per SKU is allowed, which will increase replenishments.
Scenario 1b - Increased picking productivity based on a pick path sequence goal (minimum of two pick faces per SKU was assigned).
This scenario focussed on reducing horizontal travel distance by slotting items according to a hit sequence goal (unit movement). This scenario does not take congestion into account but will keep horizontal travel distance to a minimum. A minimum of two pick faces per SKU is allowed, which will keep replenishments to a minimum.
Scenario 2a - Optimised picking quality by slotting according to density to minimise damages (limited to one pick face per SKU).
This scenario focussed on reducing damages and ensure a quality pallet built by slotting items according to density along the pick path. Only one pick face per SKU was slotted. This results in replenishments during a pick cycle.
Scenario 2b - Optimised picking quality by slotting according to density to minimise damages (minimum of one pick face assigned per SKU).
Like Scenario 2a, this scenario focussed on reducing damages and ensure a quality pallet built by slotting items according to density along the pick path. A minimum of two pick faces per SKU was slotted. This ensures that replenishments are limited to less than once daily, avoiding replenishments during a pick cycle.
Scenario 3 - Increased picking productivity based on a minimum cost sequence goal (minimum of two pick faces per SKU assigned).
This scenario focussed on reducing horizontal travel distance by slotting items according to an average cost sequence goal. This scenario does not take congestion into account but will keep horizontal travel distance to a minimum. A minimum of two pick faces per SKU was slotted. This ensures that replenishments are limited to less than once daily, avoiding replenishments during a pick cycle.
Scenario 4 - Optimised picking quality by slotting according to density based on a pick path sequence goal. A second priority picking productivity was optimised (a minimum of one pick face was assigned per SKU).
This scenario focussed on reducing damages and ensuring a quality pallet built by slotting items according to density along the pick path. As a second priority horizontal travel distance was minimised. A minimum of two pick faces per SKU was slotted. This ensures that replenishments are limited to less than once daily, avoiding replenishments during a pick cycle.
Outcomes
The different scenarios were used to determine the best slotting strategy to implement to ensure the daily picking targets will be met.
Picking productivity was the main priority when choosing the optimal scenario.
When analysing the results and comparative reports, as indicated in Figure 1, the optimal solution for this study was a scenario that would meet the picking targets as well as ensure a quality pallet built, keeping damages to a minimum.
Replenishments was also a KPI that should be avoided during a picking cycle. This was achieved by slotting more than one pick face per SKU.
Therefore, Scenario 4 was selected as the optimal scenario.
Scenario 4 indicates that the daily target would be met and even though Scenario 1a and 2a results in more productive results (192 and 187 units per hour respectively), replenishments increased by 70% for both these scenarios.
Scenario 4 also considers density that will keep damages to a minimum. Figure 1 indicates the KPI’s for all the scenarios analysed.
Scenario 4 was implemented by means of move lists that were generated by the Optislot® software to enable the client to re-slot the warehouse in a staged manner.
2021 data was also analysed by importing a new item list with the 2021 additional SKU’s as well as considering future order data. This gave the client a good indication of how the model will perform in the next year.
Challenges Addressed
The slotting solution addressed the following operational challenges:
High demand. High picking targets should be met hourly
Running out of warehouse space
Replenishing during a picking cycle
Management demands to increase productivity without new equipment purchases
Existing space not fully optimised
Product damage is impacting customer satisfaction
Picking travel distance and congestion increase as new products are shoved into first available space without pick path consideration
FIGURES
Figure 2: Comparative report for all scenarios analysed