Blog - Business Environment
Overcoming Data Challenges in the Mining Industry - Strategies for Success
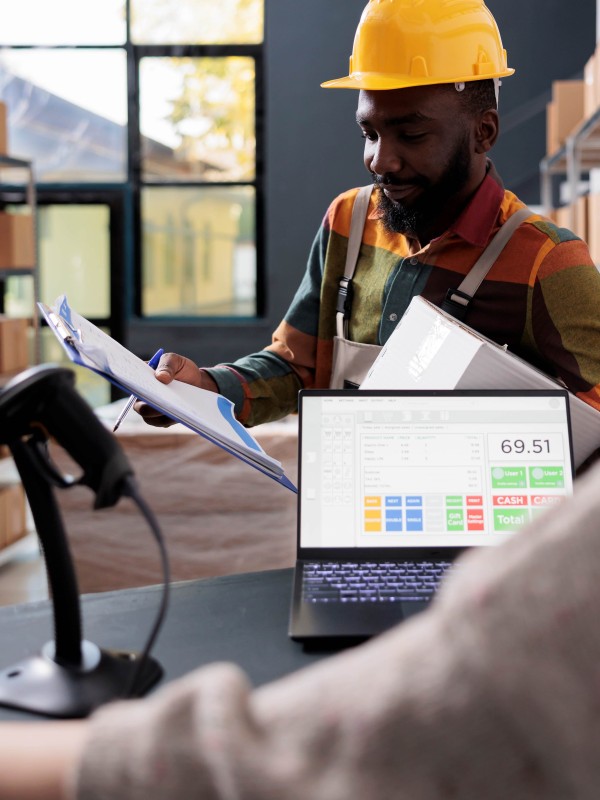
Embracing Digital Transformation in Mining
McKinsey's study of 140 analytics implementations across multiple mining operations highlights the potential of advanced analytics in the industry. For instance, an advanced-analytics approach improved gold output by optimising the leaching extraction process based on extensive data analyses, boosting the mine’s average yield by 3 to 4 percent within three months.
Despite these benefits, the journey to digital transformation in mining is not without its challenges. This article explores some of the biggest data challenges in mining and outlines strategies for overcoming them.
Environmental and Human Factors
The primary hurdle to digital transformation in mining has always been the challenging environment. Mining operations function in remote, harsh conditions with significant safety concerns and skills shortages, which have historically slowed down digitisation. However, advancements in IT infrastructure, automation, and IoT devices are breaking down these barriers.
The next major hurdle is the human factor. While data is now abundant and readily accessible, it is often used in silos. When data is used to report on other departments' performance, its credibility is frequently questioned. To overcome this, top management must support a data-driven culture, empowering employees to take ownership of their data and reporting. Employees should see data not as a punitive tool but as an empowering truth. This cultural shift is crucial and requires years of perseverance. Successful data adoption often results from tasking objective improvement teams, who have no departmental bias, as data champions.
The Importance of Robust Architecture
Digital skills are essential in today’s working environment. Anyone can create a spreadsheet or a Power BI report, but stable data workflows require more. Is your data system immune to capturing mistakes? Does it scale to large data sets and multiple sources? Are your dashboards universally accessible and seamlessly updated? A solid data architecture can answer these questions.
A robust architecture requires skilled architects and effective communication between IT and business units to ensure a collaborative approach. Aligning IT teams to support a scalable data platform is critical as data demands grow.
Ensuring Data Quality
Data quality is a notorious issue in mining due to unknown requirements and disparate data-capturing systems. Data is often collected without a clear purpose, leading to capturing errors. Understanding "quality" is crucial; it must be specified upfront and cannot be inspected in later.
Data quality can be measured through validation (asking if the data is right) and verification (asking if it is the right data). Following a good business analysis process and ensuring necessary measurement tools are in place, such as reporting tools to indicate capturing or ETL errors, are key steps to maintaining data quality.
Managing System Performance
As dependence on data increases, system performance becomes a critical issue. In mining, focus areas in the value chain continuously shift, leading to rapid changes in report usage and data demand. This haphazard usage growth can create system bottlenecks.
To manage performance issues, consider your infrastructure’s licensing strategy, and ensure monitoring and performance reports are available. Usage reports help understand data utilisation frequency, and rules for setting up refresh schedules can spread data pipeline loads effectively.
IT- OT Convergence
The convergence of IT and operational technology (OT) in mining introduces technical complications and confusion over departmental responsibilities. Traditionally, plant engineers have managed systems and data reporting. To address this, businesses need to evaluate IT capabilities and align them with business strategies.
A strong IT department with the necessary business analysts, developers, and operational teams can act as the glue, ensuring data works for the organisation. However, other departments must also take ownership of their data.
Conclusion
The digital and data revolution has finally arrived in the mining industry, bringing numerous data challenges, including environmental, human, architectural, quality, performance, and IT- OT convergence issues. Overcoming these challenges requires strategic approaches at every level. Employing the right strategies can unlock significant benefits, making mining operations more efficient, profitable, and sustainable.
References:
1. McKinsey & Company. (n.d.). [Study on analytics implementations in mining operations] (https://www.mckinsey.com/industries/metals-and-mining/our-insights/how-digital-innovation-can-improve-mining-productivity).
For more insights on leveraging data in the mining industry, contact us at This email address is being protected from spambots. You need JavaScript enabled to view it.