Blog - Operational Technologies
AI Adoption by companies: what are the challenges and how to overcome them?
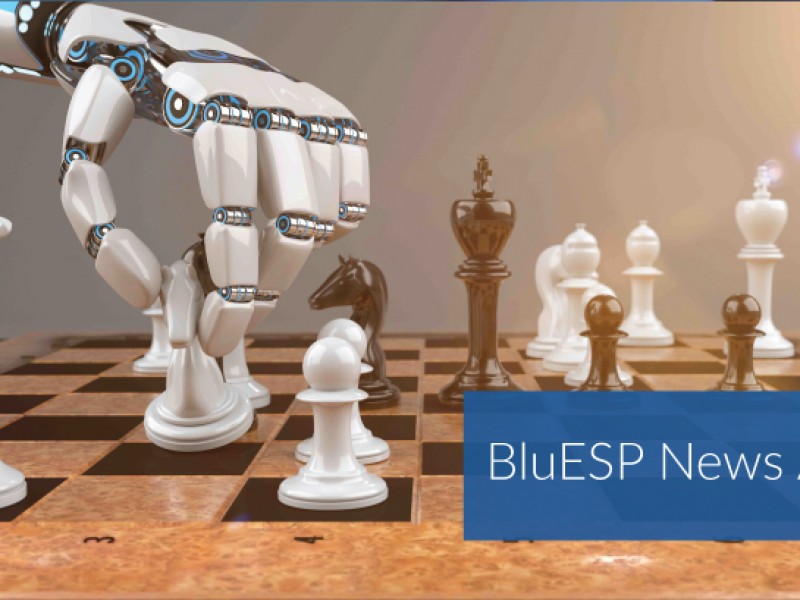
For the purpose of this article, Industrial AI is defined as:
a systematic, collaborative and integrative discipline that focuses on developing, embedding, and deploying machine learning algorithms, as fit-for purpose, domain-specific, industrial applications, that offer sustainable business value.
Forces Driving the Adoption of Industrial AI
Let’s take a closer look at the forces driving the adoption of Industrial AI.
Firstly, industrial organisations are increasingly shifting their focus to understand how AI can solve domain-specific challenges. This process links AI solutions to tangible business outcomes, bolstering the case for more widespread adoption of industrial AI
Secondly, one of the key barriers to the adoption of Industrial AI is being lowered. The bottleneck has been due to a shortage of expertise and data science skills.
Targeted and embedded Industrial AI applications are combining data science and purpose-built software. These fit-for-purpose, embedded AI applications allow users to tackle domain-specific functions with greater accuracy and across the entire industrial asset’s lifecycle. This functionality is circumventing the need for Data Science Experts.
Thirdly, is the recent trend whereby organisations are realising the value of Data as a critical component for creating actionable insights. With this in mind, organisations are capturing and storing as much data as possible. The challenge now lies in strategically collecting high-quality data and deploying High ROI data management strategies. Industrial AI is emerging as the key to unlocking the full potential of Industrial Data Sets.
The last and biggest driver of Industrial AI is the increases in productivity that capital-intensive industrial processes are reporting. Industrial AI is allowing asset optimisation without the need for expensive Data Science intervention. This is allowing industrial organisations to achieve unprecedented levels of safety and productivity in their processes. Resulting in new insights, continuous improvements, and better decisions.
Five Degrees of Readiness
Companies who wish to adopt Industrial AI and maximise its benefits will need to achieve states of readiness in the following five dimensions.
Firstly, companies need an action plan mapping Industrial AI to business goals, data objectives, and KPI’s. This is needed to incorporate Industrial AI into your current digital transformation strategy. Single-use AI applications need to make way for overarching plans that incorporate Industrial AI into business goals.
Secondly is that companies need to realise that Industrial AI’s capabilities are leveraged in relation to the quality of the Data. Garbage In results in Garbage Out. To overcome the affliction of bad data, companies need to construct data pipelines and data collection strategies across their entire organisations to ensure consistent results.
Thirdly, Future Proofing your Industrial AI Investment is achieved by laying the optimal groundwork for AI readiness. To this end, companies need to collaborate broadly across industrial environments, realising that software, hardware, architecture, and personnel form the basis upon which your industrial AI Infrastructure is built. The optimal Industrial AI infrastructure should maximise ROI by accelerating time to market, have built-in scalability, and harmonise the AI model across the lifecycle of all applications.
Fourthly, companies need to recognise that roles, skills, and training are critical. You will need the right people to implement and execute your AI strategy. This will require targeted efforts to cultivate the skills and mindset needed, while also investing in retraining significant portions of the workforce to ensure successful implementation.
Lastly is that ethical and responsible AI use is based on openness and transparency that keeps everyone in the loop regarding new developments. Clear channels of communication, reliable process documentation, and stakeholder alignment will ensure greater cooperation and result in a synergy that creates a sum that’s greater than its parts.
The above should only be viewed as guidelines, but with the emphasis always falling on the holistic implementation of an AI strategy.
Three Use Cases for Bringing your AI strategy to Life
Before embarking on implementation, your organisation needs to identify the business problems, corporate objectives, and strategic goals that Industrial AI must solve and achieve. Companies who are serious about using Industrial AI to drive business value can take inspiration from the following three business cases.
Predictive maintenance is currently the largest use case for industrial AI, constituting 24% of the total market for industrial AI in 2019. Predictive maintenance detects deviations from normal patterns of behaviour and can prescribe detailed corrective actions to mitigate and solve problems. Inasmuch, it is highly effective in optimising output and reducing downtime.
The second use case shifts the focus to quality and reliability. Where quality is indicative of how well the primary function is performed while quantity relates to how long the original quality is maintained over time. Both of these are significant measurements that, when fully and accurately understood, result in saving time and money.
The third use case is where process optimisation leverages advanced Machine Learning methods in conjunction with sophisticated deep learning neural networks to infer information and intelligence from diverse data sources, assets, and processes. This allows organisations to identify and mitigate inefficiencies, enabling direct impacts on productivity.
The three use cases outlined above present clear and concise starting points for organisations wishing to re-invigorate, re-design, or implement an Industrial AI strategy. The sooner you get started, the sooner you will reap the benefits.