Blog - Operational Technologies
Beyond predictive maintenance in mining
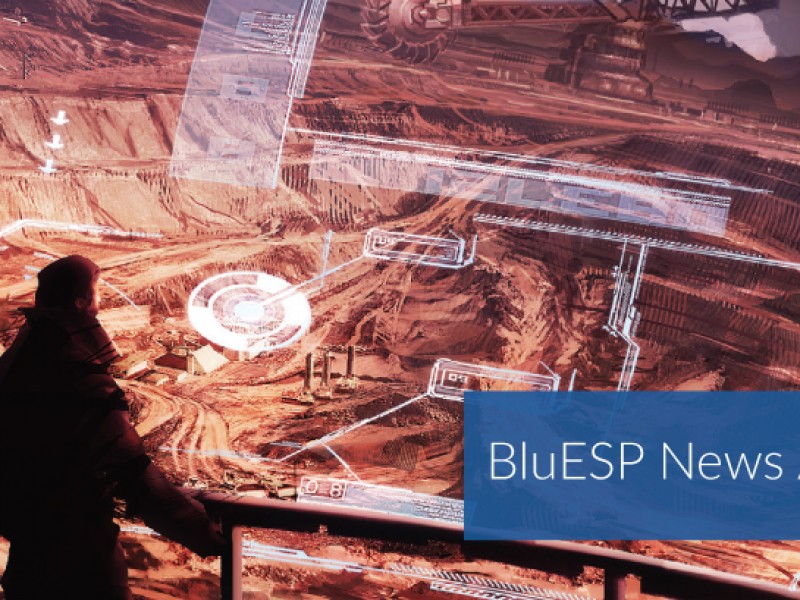
Some mining companies try to avoid these losses by stocking extra spare parts or through over-maintaining equipment. But these solutions often only add more financial burdens in CAPEX and maintenance costs. But what if all of this extra cost could be avoided? What if the machines could warn their operators about impending breakdowns days, even weeks in advance? And what if this was possible without further instrumenting existing equipment? Real-time condition-based monitoring of assets is an attractive solution for mining companies to minimise their unscheduled downtime and improve reliability of critical equipment. While many companies already have systems in place to record maintenance and reliability data with the help of sensors and control systems, collecting the data is only part of the story. The success of an asset monitoring program depends on how well this data is analysed, filtered and categorised to enable accurate predictions of impending failures. Focusing on the behaviour of an asset only tells a small portion of the story. To fully predict failures, the trends of the entire process must be considered as a whole. Aspen Mtell® is an application that uses machine learning to monitor and analyse data across the entirety of the process, from any equipment or system in real-time to detect anomalies and predict failures, and their causes, before they occur. Using Aspen Mtell®, operators can address any abnormal behaviour of the equipment before it becomes a serious problem, saving a lot of money to the business. Michel Bachoir, Principal Solutions Consultant at Aspen Tech, says Aspen Mtell® goes beyond a predictive maintenance (predictive failure detection) tool to offer a prescriptive solution (diagnosis about the causes).
“Most condition monitoring products can only speculate on time-to-failure and require further analysis to identify the problem. On the other hand, Aspen Mtell® can detect precisely how and when the failure will occur by analysing anomalies and comparing them against historical failure patterns. The maintenance team can then take action to mitigate or prevent the failure altogether,” he explains.
Bachoir elaborates on the prescriptive maintenance approach with the help of an example. “If you have vibration monitoring sensors set up on a rotating equipment, you can detect when the vibrations escalate beyond set limits. In all these cases the data need to be further interpreted to identify the real problem. Vibration can be caused by several factors such as wear on the bearing, an impeller/rotor imbalance or a loose mounting bolt. Additionally, its cause and impacts can be seen upstream and downstream of the equipment in the process data; but this is not indicated with vibration sensors. In this case, predictive maintenance cannot tell you exactly what the problem is without further analysis and validation. By using multivariate analysis – which looks at mechanical and process variables – Aspen Mtell® looks at signatures that the human eye cannot see way before a sensor reading reaches its set limit and can tell you exactly what the incoming problem is. “With vibration monitoring alone, by the time the alarm sounds, the equipment may be already damaged and you may have a very short timeframe to repair or replace that equipment.” This precision in predicting failures is rooted in Aspen Mtell’s® smart algorithms. Aspen Mtell® is flexible and can integrate with most OEM and third-party condition monitoring systems, as well as with existing process and mechanical sensors. Once set up, the system uses software elements – known as agents – to identify signatures for both normal and failure behaviours. When any failure behaviour is detected, Aspen Mtell® agents send alerts to users, prescribing appropriate maintenance activity based on the machine’s historic behaviour. The operations and maintenance teams have an extended warning of the potential for failure and can make decisions to remediate the failure in the most cost-effective way or adjust production. “Moreover,” says Bachoir, “Each time a new anomaly is detected that is not historically recorded, the maintenance team can investigate the cause and categorise it either as a new normal behaviour or a new failure signature.” While in the traditional preventive maintenance approach it is quite common for mining companies to schedule regular maintenance sessions at fixed intervals, Bachoir says Aspen Mtell’s® prescriptive approach allows the maintenance teams to manage repair or replacement choices over the lifecycle of their assets. “Scheduled maintenance shutdowns are often planned based on the expected life of the equipment. But in practice the equipment’s useful life depends on so many other factors such as process variations or equipment defects.” “So in the preventive model, the equipment is still prone to breakdowns. On the other hand, stopping the machines at fixed intervals for regular maintenance schedules is also a waste of valuable time and resources, as in many cases the full life of the equipment is not utilised,” says Bachoir. The increased reliability with asset monitoring allows plant managers to use their resources more efficiently. “For critical operations, plant managers tend to have standby equipment in place to deploy in case of an emergency. Using Aspen Mtell® allows them to avoid such emergencies by improving the reliability of the existing equipment. Having the equipment run at optimal efficiency and energy consumption also results in higher overall yield.”
While some asset monitoring systems require expert skills and knowledge of industrial equipment, Bachoir says Aspen Mtell is far simpler to implement and offers faster payback. “Aspen Mtell is very user-friendly. It requires minimal input from the end user and is very fast to implement. After a quick initial set up, the asset owners are trained to fine-tune the system and improve the agents as they go along,” he explains. Combining the Aspen Mtell solution with the client’s domain knowledge is a key driver for success, as it minimizes any delay between a notification, investigation and remediation. “The beauty of Aspen Mtell’s algorithm is that once set up, it continues to learn and adapt to the system. This means more and more reliability and peace of mind for the asset owners.”
Having an efficient asset monitoring system also means more safety for the personnel, according to Bachoir.
- It provides a stable operation with well-planned work that is less likely to miss hazards. Planned work is not only more efficient but also safer.
- Plant operators can also minimise the number of inspections that would expose personnel to the risks of interacting with the equipment.
- Aspen Mtell also facilitates better remote monitoring of the equipment so that fewer personnel are required to be present on the site.
- Aspen Mtell provides an alternative to further instrumenting equipment currently monitored mainly via visual inspection.
- If an abnormal behaviour is detected in a machine or component, the supervisors can carefully assess the situation before sending a staff member to inspect the machine.
- Research evidence indicates that about 80 per cent of industrial accidents are caused by such human factors as fatigue and anxiety.1 A stressful environment where the norm is reactive work can also increase the likelihood of accidents. Timing is also crucial, well planned work can be executed during adequate hours of the day rather than breakdowns that can happen during the last hours of a shift or during the night where a lack of attention can increase the probability of accidents.
Joint venture article with Aspen Tech and Australian Mining volume 112/5 | JUNE 2020