Blog - Operational Technologies
Navigating Artificial Intelligence and Machine Learning
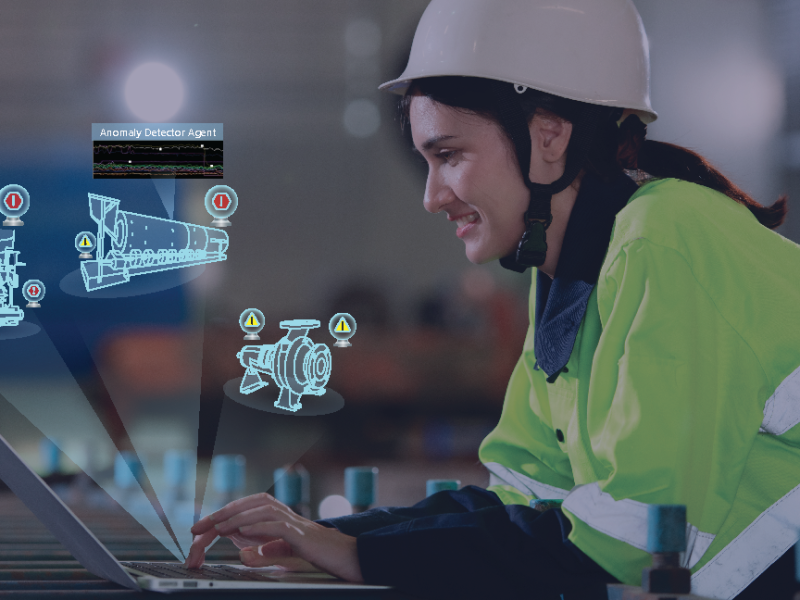
Artificial intelligence and machine learning algorithms have the potential to usher in groundbreaking transformations in manufacturing operations. However, it’s crucial to recognise that there is no one-size-fits-all solution. Implementing AI and ML can be a complex and expensive journey, and simpler alternatives may suffice in many cases. To help you determine the right path for your facility, we’ll explore how to strategically deploy advanced analysis and artificial intelligence and machine learning technology.
Assessing Your Technology Mix
When embarking on the AI and ML journey, it’s essential to begin with a comprehensive evaluation of your asset infrastructure as a whole, rather than focusing solely on individual pieces of equipment. Aspen Tech, a seasoned player in the asset performance management (APM) market, provides insights on how to choose where to apply advanced analysis when considering AI/ML applications.
Selecting the Appropriate Technology Solutions
Traditionally, vibration analysis has been a standard practice for asset monitoring in manufacturing. This data can be leveraged by data scientists to make predictions, but it can also be utilised for rules-based monitoring. For instance, if an asset’s vibration readings fall outside a specific range, it can trigger a work order. The choice between these monitoring techniques depends on the specific needs of your operation. Koos du Toit, APM Manager at 4Sight Operational Technology, highlights the importance of having a diverse toolkit for analysis. The key is to match the right analysis with the specific problem you aim to solve. Instead of overcomplicating solutions with deep algorithms and analysis, simplicity should be a guiding principle for effective APM.
Considering Assets as Part of a Larger System
Understanding that each asset plays a unique role in your infrastructure hierarchy is crucial. AI technology can effectively use historical data on asset failures to identify patterns and address issues pre-emptively. Different types of agents and monitoring techniques, including rules-based, condition-based, and AI/ML-based approaches, are deployed to tailor solutions to the specific needs of each asset.
Choosing Assets for Deeper Analysis
Selecting the right assets for AI/ML deployment is pivotal. To determine which assets require deeper analysis, ask questions like: Which assets cause the most problems in your facility? Which failures have the greatest impact on production? Focusing on the most expensive assets might not always yield the best results, as these may already receive extensive maintenance. Taking a holistic approach and considering the entire production process can provide a better understanding of how to improve maintenance practices.
Determining the Data Requirements
To initiate AI and ML technology, you’ll need some information about asset failures, often sourced from an enterprise asset management
(EAM) system. While there’s no fixed timeline for collecting this data, the quality of the data matters more than its quantity. Machine learning relies on training models, and the more quality data you have, the better your model’s performance will be.
Incorporating End-User Expertise
When failure data is limited, monitoring information from sensors can provide valuable insights. Anomaly detection can help identify issues based on deviations from typical sensor patterns. In such cases, subject matter experts can play a crucial role in identifying the root causes of anomalies and facilitating the decision-making process.
Balancing Throughput and Quality
APM technology can assist manufacturers in achieving a delicate balance between product throughput and quality. By adjusting process parameters with the help of technology, manufacturers can extend the life of older equipment without compromising quality. This requires effective communication between operations and maintenance teams.
Optimising Maintenance Schedules
AI-powered APM technology can consider various factors, including equipment data, production goals, scheduling demands, and supply issues, to determine the optimal timing for maintenance. The more information you feed into the system, the more it can optimise maintenance schedules based on your specific operational requirements.
Setting Business Goals
A successful APM project starts with a kickoff meeting involving the digital/IT team, maintenance and reliability, and operations. Business goals should be defined in alignment with operational needs. This may involve a vision workshop to chart the future direction and desired outcomes. Frequent check-ins and status reports help ensure that all teams remain aligned and that the project is on track.
Four Sight emphasizes collaboration with customers, aiming to understand their usage of the software and gather feedback for future improvements. This approach creates a continuous cycle of innovation, enhancing the software’s capabilities based on real-world applications. In conclusion, adopting AI and ML in manufacturing operations requires a thoughtful, strategic approach. By tailoring your technology mix, considering assets as part of a larger system, and involving end-user expertise, you can unlock the potential of these technologies while ensuring alignment with your business objectives.