Whitepapers - Operational Technologies
Hybrid Modeling: AI and Domain Expertise Combine to Optimise Assets
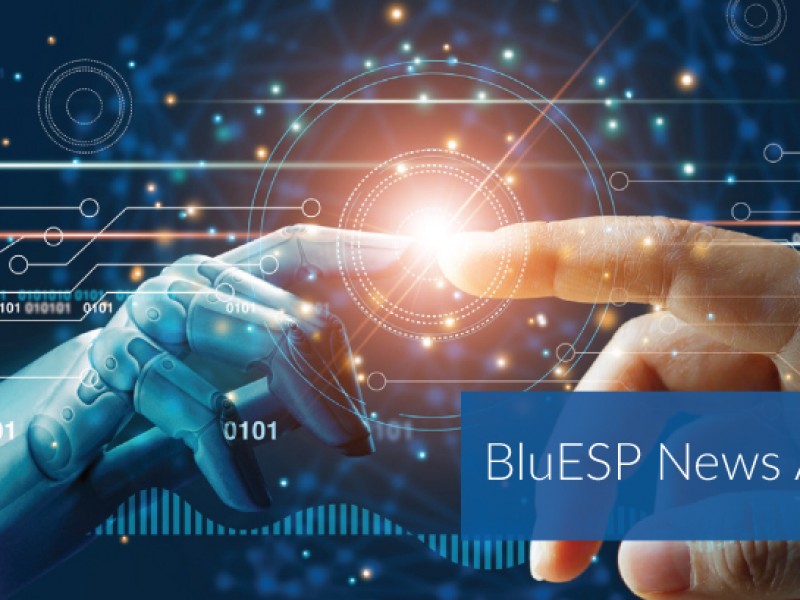
Executive Summary
Aspen Technology, Inc. (Aspen Tech) has invented a method for integrating first principles-based process simulation models and domain expertise with AI and analytics algorithms. The resulting application software is a hybrid modelling system that achieves more than either first principles modelling or AI could alone. First principles models are well established for their accurate modelling of chemical and hydrocarbon processes. Aspen Plus® and Aspen HYSYS®, the world’s premier chemical process simulation systems, have an accuracy and predictive capability validated, relied on, and improved on over four decades of use by industry, researchers, and scientists. Their models and first principles relationships are based on hundreds of years of experience by the world’s best process engineers and operators, comprising expertise from Aspen Tech’s R&D teams, Aspen Academy’s university researchers and customers who have provided inputs and contributions. To get the final several percentage points of accuracy out of these models, plant data is employed to calibrate these first principles models to observed plant conditions and performance. Effective model calibration requires considerable expertise and experience today, despite the many workflow automation improvements Aspen Tech has provided. AI and machine learning are rapidly emerging as tools that can greatly accelerate the ability to employ plant data both to calibrate first principles models and to create data-based models of phenomena and processes quickly. AI has the potential of lowering the expertise bar towards modelling process systems, but it must be combined with domain expertise to create the real-world guard-rails that make it work safely, reliably and intuitively. Hybrid models combine AI, first principles and domain expertise to deliver a comprehensive, accurate model more quickly without requiring significant expertise. Machine learning is used to create the model, leveraging simulation, plant or pilot plant data, while using domain knowledge including first principles and engineering constraints to build an enriched model without requiring the user to have deep process expertise or be an AI expert. This next generation of solutions democratizes the application of AI within hybrid models to optimally design, operate and maintain assets – deploying them online and at the edge. AI and machine learning allow us to build a model analysing a broader set of data while leveraging advanced data science techniques for model prediction. When combined with engineering principles and domain expertise, the models can be built and maintained more quickly than traditional methods without requiring significant user expertise.
With hybrid models, users can model processes and assets that cannot easily be modelled with first principles alone. Examples include:
- batch processes, which can be too varied to systematically model
- fluidized bed processes with complex chemical and fluid behaviour
- bio-process reactors and fermenters • complex refining units
Users get the accuracy of empirical models and the strength of first principles models, leveraging the power of AI paired with domain expertise, to create a more predictive model faster and with
less experience required than ever before. Hybrid models provide a better representation of the plant, which keeps the model more relevant over a longer period of time. This reduces the barrier to entry for using modelling for asset optimization by requiring less effort and expertise. With the models in place, the connected worker becomes free to perform higher value-added and strategic work. Aspen Tech will be deploying hybrid modelling capabilities across its existing software suite through a model alliance approach, which synchronizes fit for purpose models in different functional areas needed to safely, reliably, sustainably and profitably operate an asset. An example of the model alliance is the use of reduced order unit models in planning, dynamic optimization and online equipment monitoring, all derived from the same root refining unit operating data set and simulation model, achieving closed-loop production optimization.
Current Business Challenges:
The process industry faces unprecedented uncertainties and macro-economic threats. Process industry leaders face unparalleled volatility in all phases of their business. External factors including hydrocarbon price turbulence, changes in remote working needs and supply chain interruptions are making change inevitable for process manufacturers, from the smallest to the most global. Ad-dressing challenges ranging from changes in feedstock price and demand to society’s drive towards sustainability, organizations must weigh complex trade-offs. Software technology, and in particular AI, is widely viewed as one of the primary tools available to equip organization to thrive amid these challenges.
Market Volatility and Energy Transition
A trio of external forces are forcing continued volatility and turbulence on energy and chemical companies globally. The global market supply and demand shock and economic recovery we are entering, the societal drive for energy transition and carbon zero industry, and the social contract driving for zero casualties and environmental incidents all have a massive impact on industry executive teams’ thinking.
Process industry companies are fixated on flexibility, strategies for resilience in producing at unpredictable utilization factors and with extended maintenance intervals, yields and operating margins. Faster models, solving key economic units or entire sites rapidly, tuned better to plant operating conditions, answer the crucial questions needed to achieve those goals. Hybrid modelling makes it possible to model and deploy quickly — even remotely — to address dynamic market forces and asset conditions. These models become key ingredients to transform operations through the future self-optimizing plant.
The Disappearing Expert
As a generation of experts retires, process organizations face a gap in essential knowledge and a new generation of workers without the longevity to develop that critical expertise. Hybrid models, embedded with AI, address those gaps, creating immediate value for organizations and assets.
All but those enterprises with the deepest pockets need the ability to build and deploy these models without scarce and expensive experts.
Sustainability Pressures
Formidable decarbonization goals across industry will not disappear after the current economic cycle. The pressure to move towards a circular economy also creates many innovation challenges. Hybrid models provide the ability to optimize and evaluate optionality across a wide asset scope, to select the best strategies to meet these goals. Companies are challenged today to contend with the complexity that sustainability pressure imposes on their operating and strategy decisions.